blog
AI in Manufacturing: A Game Changer for Efficiency and Productivity
By Mohan S Software development App development Digital transformation AI and ML November 1, 2024
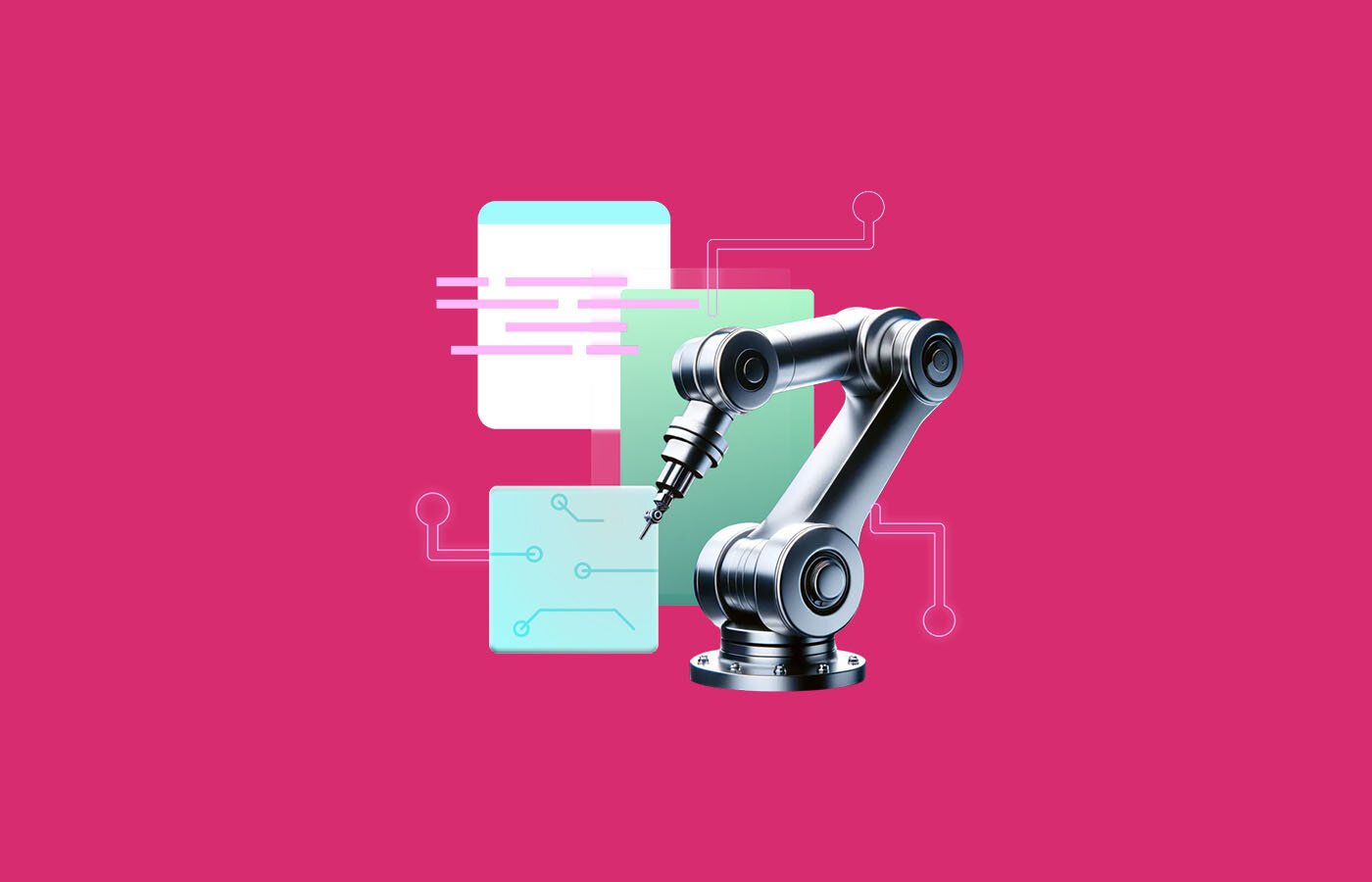
Nearly 80% manufacturers in Southeast Asia, including Singapore, are expected to increase AI investments in 2024. This trend reflects a focus on leveraging AI to optimize supply chains, enhance production efficiency, and address workforce shortages caused by an aging workforce. Companies are investing in automation and AI to mitigate knowledge gaps, with a push towards capacity-building to manage resource issues effectively.
AI in Manufacturing: Market Overview
The manufacturing industry began adopting automation as early as the 1950s. Despite this success, the sector has faced challenges in fully embracing AI. However, AI in manufacturing is expected to experience significant growth, with global spending projected to increase at a CAGR of 44.2% from 2024 to 2030.
Singapore is making progress in AI-driven manufacturing with A*STAR's launch of the AI Manufacturing Centre of Excellence (AIMfg) in 2024, aimed at enhancing the manufacturing industry through AI technology.
With companies increasingly integrating AI for enhanced productivity and reduced operational costs, the market reflects a significant shift towards intelligent automation and data-driven manufacturing processes.
Applications and Benefits
AI is central to "Industry 4.0," driving automation and enabling manufacturers to unlock the value of data. AI in manufacturing has tremendous benefits and applications:
Predictive Maintenance
AI-powered predictive maintenance systems use machine learning algorithms to monitor equipment and predict failures before they occur. This reduces downtime and extends the lifespan of machinery, resulting in cost savings and improved efficiency.
For instance, Siemens has implemented AI systems that predict component failures, reducing unplanned downtime by up to 50%.
Quality detection
AI utilizes computer vision technology for real-time defect detection during production. This enhances product quality and reduces waste, contributing to sustainable manufacturing practices.
Companies like Bosch are using AI-driven quality inspection systems that have improved defect detection rates by over 90%.
Supply Chain Optimization
It can be a life-saver in forecasting demand, optimizing inventory levels, and improving logistics. By analyzing vast data sets, AI algorithms provide insights that reduce excess inventory and improve delivery times.
For example, BMW’s AI-based supply chain management system has streamlined logistics, resulting in a 20% increase in efficiency.
Process Automation
Collaborative bots and AI systems automate repetitive tasks on production lines, increasing speed and accuracy while reducing human error.
Tesla uses AI-driven robots for assembling parts, which has led to a significant reduction in assembly time per vehicle.
Energy Management
AI optimizes energy cons factories by adjusting production schedules and operations based on real-time energy usage and cost data.
GE's AI systems, for example, have reduced energy consumption in their plants by 15%, demonstrating AI’s potential for sustainable manufacturing.
Barriers to AI Implementation in Manufacturing
Manufacturing has a massive amount of legacy technology and equipment that can't be easily retrofitted to work with the latest AI innovations. While it’s one of the main reasons why the manufacturing has been laggard at embracing AI, here are the challenges that manufacturing businesses face in integrating AI into their processes:
While high implementation costs pose a significant challenge for manufacturers looking to adopt AI, various strategies can help mitigate these expenses. By taking a phased approach and exploring government incentives, manufacturers can navigate these hurdles of AI adoption.
1. High Implementation Costs
Challenge: Small and midsize businesses may find it difficult to adopt AI in manufacturing due to the large upfront costs associated with acquiring the necessary infrastructure, technology, and trained labor.
Solution: Companies can start with pilot projects focusing on areas with the highest return on investment, such as predictive maintenance or quality control, before scaling up. Additionally, governments and industry bodies are offering grants and subsidies to support the adoption of AI technologies, which businesses can leverage to offset initial costs.
2. Security Concerns
Challenge: Since AI systems need enormous amounts of data, data security and privacy are important issues to consider. The risk of cyberattacks and data breaches increases as manufacturers connect devices and systems more frequently.
Solution: Implementing robust cybersecurity measures, such as encryption, network segmentation, and real-time monitoring systems is essential. Manufacturers should also adopt AI models that prioritize data privacy, like federated learning, which allows AI models to learn from data without it leaving its source.
3. Data Quality Concerns
Problem: Businesses trying to use AI technologies in sectors like manufacturing encounter difficulties because of the calibre of the data being used. Because AI depends so heavily on data for training and decision-making, inadequate or poor-quality data might produce poor outcomes and erroneous predictions. It might be challenging for AI solutions to function well in manufacturing settings due to disjointed data systems or irregular data collection.
Solution: Companies should prioritize data accuracy, consistency, and completeness by implementing data governance practices, regular audits, real-time data monitoring systems, and collaboration with AI providers. Training employees to handle data effectively and investing in data validation tools can also improve AI outcomes.
4. Integration with Existing Systems
Problem: Integrating AI into traditional industries like manufacturing faces challenges due to compatibility issues with existing software systems and processes, as many lack the infrastructure required for AI technologies, resulting in inefficiencies or communication breakdowns.
Solution: Businesses should spend money updating the IT infrastructure to enable smooth AI tool integration. This entails working with knowledgeable AI providers, updating out-of-date software, and putting middleware into place. By bridging the gaps between legacy software and new AI systems, custom APIs can guarantee a more seamless operation.
Conclusion
Unquestionably, AI has a revolutionary impact on manufacturing, providing innovations that improve productivity, sustainability, and quality. To truly realise AI's potential, businesses must overcome issues including high installation costs, data security, and worker skills. AI has the potential to steer the manufacturing sector towards a more inventive and efficient future with the right plans and investments.
To ensure your business leverages AI to its fullest potential and stays ahead in the manufacturing sector, partner with Buuuk. Contact us today and learn how our expert team can help you design, develop, and implement AI-driven solutions tailored to your specific manufacturing needs, ensuring your operations are optimized and future-proof.