blog
Privacy when Integrating AIs in Mobile Apps: A Developer's Perspective
By Mohan S Digital transformation AI and ML April 21, 2025
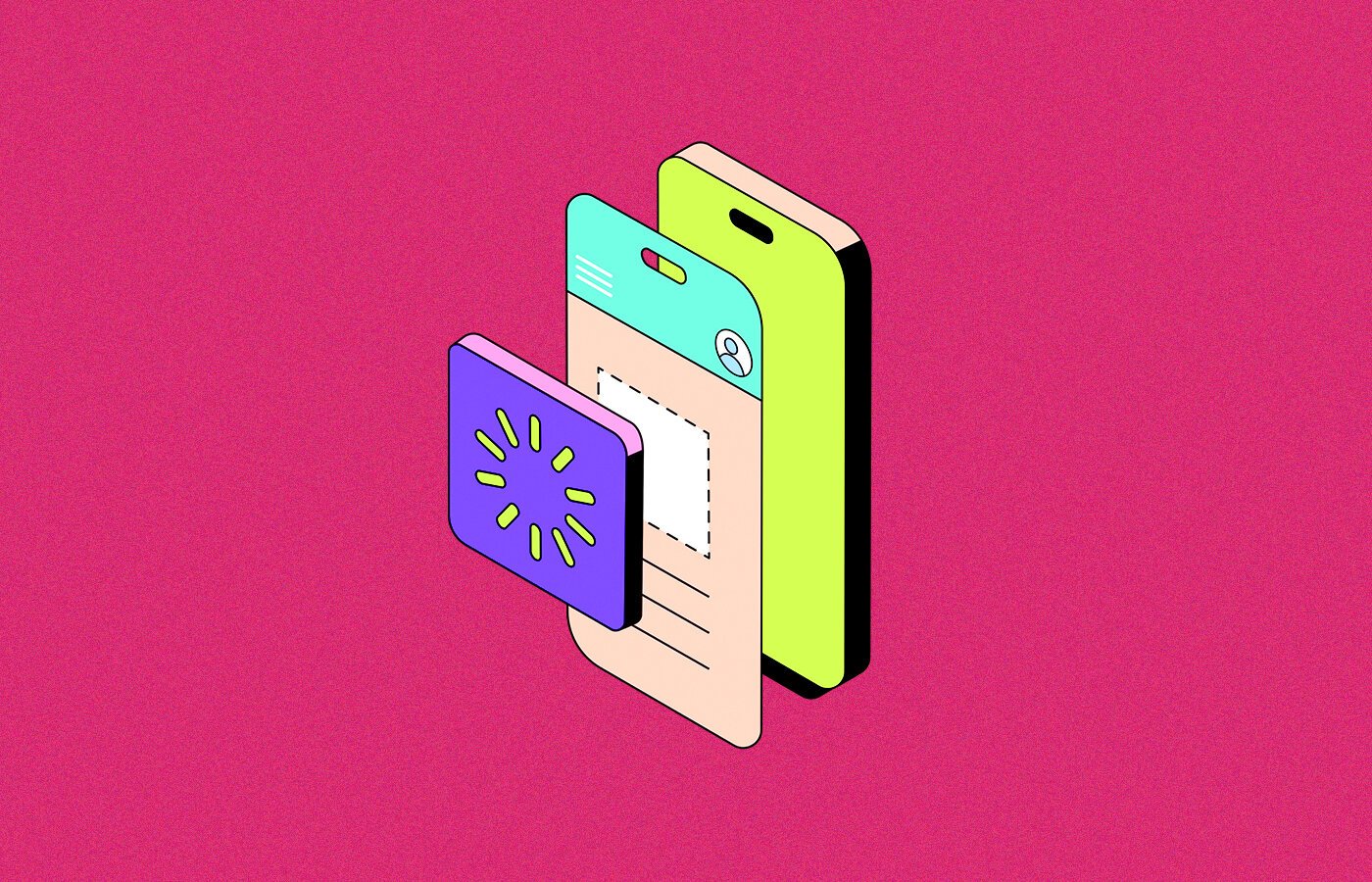
The integration of Large Language Models (LLMs) and other AI technologies is rapidly transforming mobile apps. From personalized recommendations to intelligent chatbots, AI promises enhanced user experiences. However, this power comes with the great responsibility of safeguarding user data privacy as well your clients’ and own business’. As app builders, we're at the forefront of this challenge.
The Privacy Tightrope: Balancing Innovation and Security
AI thrives on data, often including sensitive user information. This creates a tension: how can we leverage AI's capabilities without compromising user privacy?
Unlike conventional applications, LLMs, once fed data, get trained on it. Deleting the data requires re-training them, which is expensive and resource intensive.
The lack of the delete function or the complicated delete function is a critical concern in the age of privacy regulations and the "right to be forgotten."
You may like to read: Technology and the future of work
Understanding the Risks
Acknowledging potential privacy risks is crucial:
Data Leakage: Sensitive data like business client names, intellectual property, or personal data, and healthcare records can unintentionally be exposed during model training, inference, or even through application logs. Copying and pasting sensitive information into an LLM might result in that confidential data lingering within the model, potentially accessible to others.
Re-identification: Even anonymized data can be re-identified using other publicly available datasets.
Model Bias: AI models trained on biased data can perpetuate and amplify societal biases.
Data Misuse: Data could be used for purposes beyond the user's original intent.
AI "Hallucinations" and Data Regurgitation: LLMs can sometimes generate outputs containing sensitive information from their training data.
Strategies for Privacy-Preserving AI Integration in Mobile Apps
Fortunately, there are several methods to mitigate these risks.
1. Data Minimization:
Collect only the data you absolutely need. Critically evaluate the necessity of data for desired functionality.
Avoid collecting personally identifiable information (PII) when possible. Use aggregated or anonymized data when feasible.
2. Data Anonymization and Pseudonymization:
Remove or alter identifying details. This includes names, addresses, contact information, and other directly identifiable attributes.
Use random identifiers. Replace PII with unique, randomly generated IDs to prevent direct association with users.
Generalize data. Aggregate or generalize sensitive data, like converting specific addresses to city or state level.
Remember that anonymization is not foolproof. Be aware of re-identification risks.
3. Privacy-Preserving Techniques:
Differential Privacy: Add random noise to data to prevent traceability of individual records.
Federated Learning: Train the AI model on user devices without directly accessing the raw data. Only model updates are shared.
Homomorphic Encryption: Perform computations on encrypted data without decrypting it.
Secure Multi-Party Computation (SMPC): Encrypt and mask individual contributions during decentralized learning, only revealing aggregated results.
4. Input and Output Sanitization with DLP:
Implement Data Loss Prevention (DLP) APIs. DLP APIs can detect and redact sensitive data in text and images before it reaches the AI model, in logs, and in responses.
Customize data types to redact. Configure DLP to target sensitive information (e.g., credit card numbers, social security numbers, addresses).
Filter data before model input: Remove potentially sensitive information.
Redact sensitive information from logs: Prevent user-generated inputs stored for debugging from leaking data.
Sanitize AI model responses: Ensure the AI model doesn't inadvertently return sensitive user information.
5. Secure Application Logging:
Minimize logging of sensitive data. Only log information essential for debugging and monitoring.
Anonymize or redact sensitive data in logs. Use DLP or other techniques to remove PII.
Implement access controls. Restrict access to log files to authorized personnel only.
6. AI Alignment and Ethical Considerations:
Instill data ethics into your AI model. Prioritize data privacy and responsible AI practices from the outset.
Design your AI system with privacy in mind. Consider the ethical implications of your AI model and its data interactions.
Train AI models on diverse datasets to mitigate bias. Ensure fairness and equity for all users.
7. Transparent Privacy Policies and User Consent:
Clearly explain how you collect, use, and protect user data.
Obtain explicit consent before collecting or using sensitive data. Give users control and opt-out options.
Provide mechanisms to access, modify, and delete data. Empower users to manage their privacy.
Inform users about the use of AI in your app. Transparency builds trust.
8. Data Storage and Security:
Encrypt sensitive data at rest and in transit.
Use secure storage solutions. Store data securely with appropriate access controls.
Regularly audit your data storage and security practices.
9. Data Privacy Vaults:
Prevent sensitive data from entering the LLM altogether: The most practical approach to maintaining compliance is to prevent sensitive data from entering the model.
Data privacy vault isolates, protects, and governs sensitive customer data while facilitating region-specific compliance with laws like GDPR through data localization.
Vault Architecture: Store sensitive data in your vault, isolated outside of your existing systems. De-identified data that serve as references to the sensitive data are stored in traditional cloud storage and downstream services.
Tokenization: De-identification happens through a tokenization process. A token is a pointer that lets you reference something somewhere else while providing obfuscation.
Zero Trust Access: The vault tightly controls access to sensitive data through a zero trust model where no user account or process has access to data unless it’s granted by explicit access control policies.
De-identify Data to preserve privacy during model training, store sensitive data within the vault, and replace it with de-identified data. The resulting dataset is de-identified and safe to share with an LLM.
10. Developer Practices
Security audits: Regularly perform security audits to identify and remediate vulnerabilities.
Stay updated: Continuously monitor and adapt to evolving privacy regulations and best practices.
The Future of Privacy in AI
Privacy-preserving AI is evolving, and regulatory frameworks are emerging to enforce privacy standards. Laws like Singapore’s PDPA (Personal Data Protection Act) and the GDPR(General Data Protection Regulation) in Europe grant individuals rights to access, rectify, and erase their personal data, which poses compliance challenges for LLMs.
As app creators, we must stay informed and proactively adopt best practices as we innovate.