blog
Data-Driven Success: Predictive Analytics Use Cases in Modern Retail
By Mohan S Software development Digital transformation Retail October 7, 2024
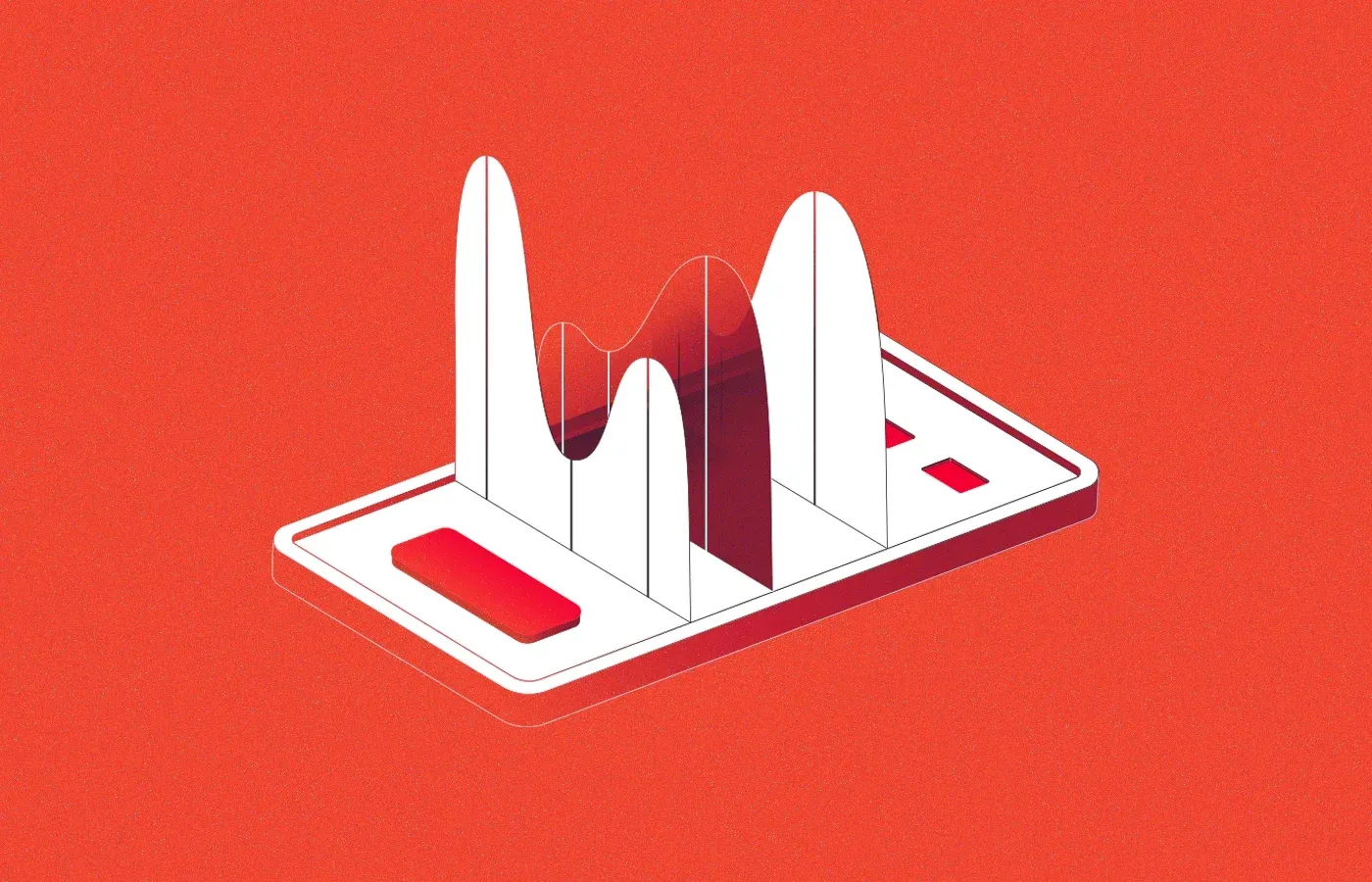
Predictive analytics in retail involves using statistical algorithms, big data integration, machine learning, and predictive models to analyze historical and transactional data, helping to forecast future outcomes.
Despite sounding like something from a scifi movie, predictive analytics has already proven its importance in retail by revolutionizing how retail businesses operate, enabling them to make informed decisions that retain customers, drive sales, reduce costs, and improve customer satisfaction.
A BCG report reveals that companies that implement predictive models bring significant financial benefits, including a 10% increase in sales, a 20% increase in profits, and a 10-20% reduction in costs. This shift has helped businesses gain a competitive edge in a rapidly evolving market.
This blog explores the critical use cases of predictive analytics in retail, supported by examples.
Examples and Use Cases of Predictive Analytics in Retail
According to a study by Dresner Advisory Services, 52% of organizations have adopted predictive analytics, which indicates a significant increase compared to previous years. This adoption reflects a growing trend among businesses to leverage data for improved decision-making and strategic planning.
Dig deeper with: No digital transformation is possible without big data
This section delves into ten key applications of predictive analytics in retail, demonstrating its impact on various aspects of the industry.
1. Inventory Management
Predictive analytics in inventory management helps retailers forecast demand and optimize stock levels. Using historical sales data and real-time analytics, stores can predict which items will be in demand during specific seasons, avoiding overstock or stockouts. An automated system could suggest reordering or halting shipments, ensuring an efficient inventory.
Checkout: The power of automation in warehousing
Example: Walmart uses predictive analytics to manage its inventory efficiently. By analyzing historical sales data and trends, Walmart forecasts demand for products during specific seasons, ensuring they have optimal stock levels, which helps avoid both overstock and stockouts.
2. Business Intelligence
By leveraging predictive analytics, business intelligence platforms in retail can uncover key insights, such as customer behavior trends and sales patterns. These insights help retailers make data-driven decisions, such as adjusting product offerings or improving customer experiences. Analyzing previous holiday season sales can guide promotional strategies for the next season.
Example: Sephora employs predictive analytics to enhance customer personalization through its mobile app. By analyzing user interactions and preferences, they provide tailored product recommendations and personalized marketing campaigns. For instance, their analytics system predicts which products a customer is likely to purchase based on their previous behavior, improving customer engagement and loyalty.
3. Customer Segmentation
Predictive analytics allows retailers to classify customers based on their purchasing behavior, demographics, and preferences. By doing so, they can tailor marketing campaigns more effectively. A retail store might use these analytics to create separate strategies for high-value customers and bargain hunters, improving overall marketing efficiency.
Example: Starbucks uses predictive analytics to segment its customer base and enhance its loyalty program. By analyzing purchase history and customer feedback, Starbucks can identify preferences and tailor rewards to encourage repeat purchases. This segmentation allows them to offer personalized promotions that resonate with different customer segments, driving engagement and increasing overall sales.
4. Supply Chain Management
In supply chain management, predictive analytics helps anticipate disruptions and optimize logistics. Retailers can use this data to forecast supplier delays and take proactive measures like rerouting shipments or selecting alternative suppliers. This ensures smooth operations even during unexpected disruptions.
Example: Amazon leverages predictive analytics for supply chain optimization by analyzing purchasing trends and customer behavior. This helps the company forecast demand for specific products, enabling efficient inventory management and minimizing delays. Amazon's data-driven approach allows them to proactively manage their logistics network, ensuring timely deliveries even during peak shopping seasons.
5. Demand Forecasting
Demand forecasting uses predictive models to estimate future sales volumes based on historical data, market trends, and external factors like weather or holidays. Retailers can better plan stock levels and marketing efforts, ensuring they meet customer demand without incurring excess costs.
Example: Zara implements demand forecasting as part of its agile supply chain strategy. By analyzing sales data and customer feedback, Zara can quickly adjust its production and inventory levels to match current fashion trends. This responsiveness allows Zara to minimize excess inventory while ensuring that popular items are available in stores, which is essential for maintaining customer satisfaction.
6. Price Optimization
Predictive analytics helps retailers dynamically adjust prices based on factors like competitor pricing, inventory levels, and consumer demand. A retailer might use these insights to offer discounts on slow-moving products while raising prices on items in high demand, maximizing profitability.
Example: Zalando, a leading European online fashion retailer, uses predictive analytics for price optimization by analyzing customer preferences, demand patterns, and inventory levels. This approach enables Zalando to adjust prices based on real-time data, helping to clear out excess inventory while maximizing sales of trending items.
7. Fraud Detection
Predictive analytics plays a crucial role in detecting and preventing fraud in retail. By analyzing transaction data and identifying unusual patterns, retailers can flag potential fraud cases in real-time. If a sudden surge in high-value transactions occurs, the system could alert fraud prevention teams before any loss occurs.
Example: PayPal employs predictive analytics to enhance its fraud detection capabilities. By analyzing transaction behaviors, the platform can identify irregular patterns that may signify fraudulent activities. For instance, if a user suddenly makes a series of high-value purchases from an unusual location, PayPal's algorithms can trigger alerts and potentially freeze the account to prevent unauthorized transactions.
8. Marketing Campaigns
Retailers use predictive analytics to enhance the effectiveness of marketing campaigns. By analyzing customer behavior, such as browsing history and purchase frequency, retailers can deliver personalized marketing messages. A customer who frequently buys athletic wear may receive promotions for new sports collections.
Example: Netflix employs predictive analytics to personalize content recommendations for its users. By analyzing viewing habits and preferences, the platform can suggest shows and movies that align with individual tastes. This strategy not only keeps users engaged but also drives subscription renewals, showcasing the effectiveness of targeted marketing.
9. Employee Performance and Workforce Optimization
Predictive analytics improves retail employee performance by analyzing sales data, customer interactions, and employee schedules. Retailers can identify high-performing employees and replicate successful practices across teams.
They can utilize predictive analytics to evaluate employee performance during peak shopping seasons. By assessing sales figures alongside employee schedules, they can identify top performers who excel under pressure and develop strategies to replicate their success across other teams.
Example: Walmart has effectively utilized big data analytics to enhance employee performance across its stores. By analyzing a range of data points, such as sales figures, customer interactions, and employee schedules, Walmart can identify high-performing employees and best practices. This allows the company to tailor training programs to address skill gaps, ensuring that employees are better equipped to meet customer needs.
10. Enhanced Customer Feedback Mechanisms
Predictive analytics improves customer feedback by leveraging data from social media, online reviews, and surveys. Retailers can identify areas for improvement and respond to customer concerns before they escalate.
This data-driven approach allows retailers to segment customer feedback based on demographics or purchasing behavior, enabling personalized communication strategies and tailored product offerings.
Example: Nike utilizes predictive analytics to analyze customer feedback from various channels, including social media, customer reviews, and surveys. By identifying trends in customer sentiment, Nike can quickly address concerns regarding product quality or availability. For example, if they notice a recurring issue with a specific shoe model, they can proactively engage customers, implement design improvements, and communicate these changes through targeted marketing campaigns. This responsiveness has helped enhance brand loyalty and customer satisfaction.
These examples show how predictive analytics transforms retail by driving smarter decision-making across different areas of the business.
Conclusion
Predictive analytics is reshaping the retail industry by providing valuable insights into customer behavior, inventory management, pricing strategies, and supply chain operations. As more retailers adopt data-driven decision-making, predictive analytics will continue to play a pivotal role in the future of retail, driving innovation and competitiveness.
Unlock the full potential of your business with Buuuk's innovative solutions! Learn more about our services here.